Location
Houston, Texas, United States
s87610281@gmail.com
Languages
Mandarin, English
Hello! This is Zheng-Chen.
I'm .
About Me
I earned my Bachelor's degree from National Yang Ming University, Taiwan, Taipei, with a biology major. I am currently a trainee in Dr. Irina Larina's Lab at Baylor College of Medicine, Houston, Texas. My research is focus on the quantitative analysis of the sperm dynamics and investigation of reproductive system. In my spare time, I enjoy programming and solving algorithm problems.
10+
Projects
Completed
1
Papers
Published
5+
Conferences
Attended
200+
Images
Acquired
Programming skills
HTML5
CSS
Javascript
Python
C++
Git
Projects
Quantitative analysis of spermatozoa hyperactivation state
Aim:
The purpose of this project is to establish a quantitative model based on the spermatozoa trajectories which can identify if spermatozoa are hyperactivated at specific imaging intervals.
Introduction:
The method of identifying the state of spermatozoa in a 2D slide under microscopy is well-developed for a long while and widely used in reproductive research. This method must extract the spermatozoa from the epididymis and load them into a chamber, which means spermatozoa were imaged in vitro environment. However, the actual reproductive events happen in the female reproductive tract and the physiological environment there is largely different from in vitro experiments. The in vivo investigation is highly desired.
* The figure shows waveforms of flagellum ------>
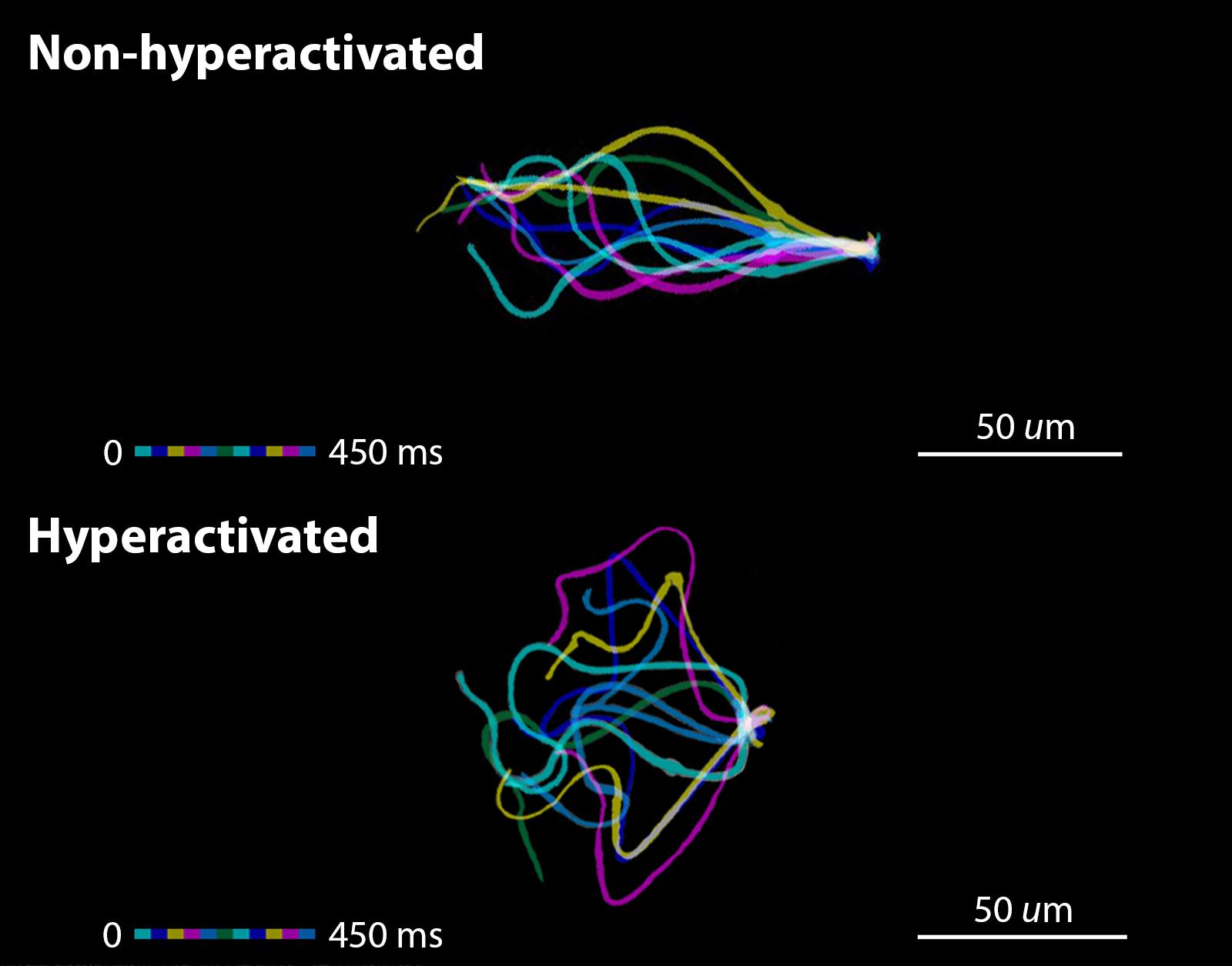
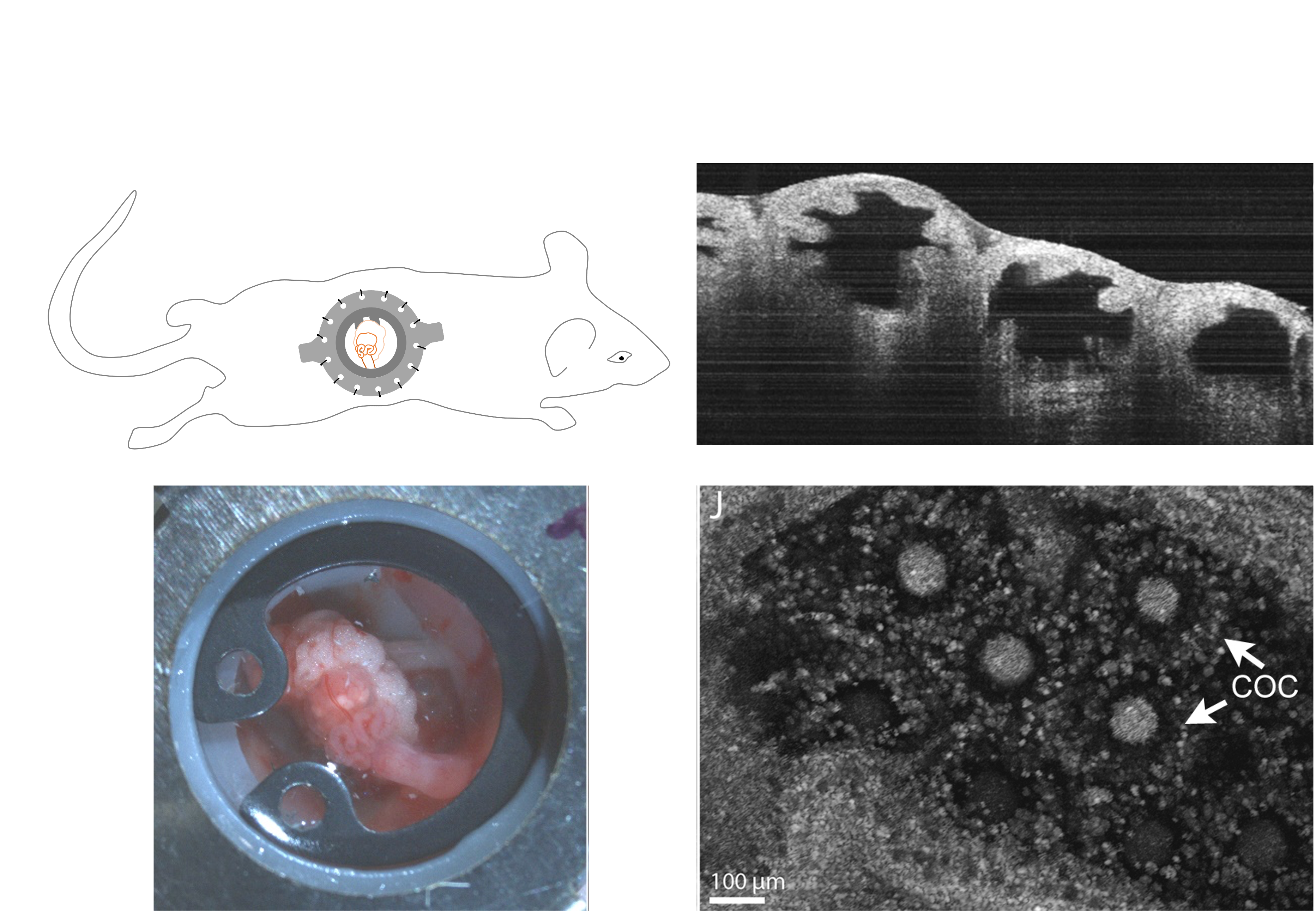
^^^^^^^^^^^^^^^^^^^^^^^^^^^^^^^^^^^
* In-vivo OCT imaging and mouse surgery
Take advantage of our unique imaging technology, our lab can acquire real-time in vivo images. The acquisition of in vivo images is an extremely difficult process due to the uncertainty of the mouse. It is impractical to gather thousands of in vivo trajectories to analyze. However, extracting spermatozoa in vitro and tracking sperm’s behavior is more feasible. The goal of this study is to collect tons of trajectories and establish a model in vitro to solve the binary classification problem in vivo. The model is expected to predict the state of specific spermatozoa is either hyperactivated or non-hyperactivated.
For the data acquisition, spermatozoa from the same mouse were divided into two groups. One of them was imaged immediately as non-treated control. They are expected all be non-hyperactivated. Another group was induced by hyperactivation treatment, which will induce a portion of spermatozoa undergoing an essential transition to become hyperactivated spermatozoa. In other words, we got a mixture of non-hyperactivated and hyperactivated spermatozoa from the hyperactivation-treated group and the goal is to classify them.
* Parameters using to identify hyperactivation state ------>
The coordinates of spermatozoa movement were recorded, and multiple features were extracted as parameters to evaluate the hyperactivation state comprehensively. Three different parameters, Linearity (LIN), Straightness (STR), and Wobble (WOB) are derived from velocities (VCL, VSL, VAP) calculated in three different ways. Another parameter, the Standard Deviation of Direction Variation (SDofDV), was used to represent the dispersion of direction change.
* Flowchart of ensemble classification model ------>
Before building the unsupervised classification model, four features (SDofDV, LIN, STR, WOB) of each trajectory in the mixed pool were performed by Principal Component Analysis for better data visualization. The idea of this ensemble classification model came from the well-known machine-learning model – Random Forest. I resample 80% of trajectories with replacement to generate 2000 bootstrapped datasets. The k-mean clustering algorithm was applied to these bootstrapped datasets to build 2000 weak classifiers. These weak classifiers acted as voters to determine the hyperactivation state of an unknown spermatozoa through majority voting.
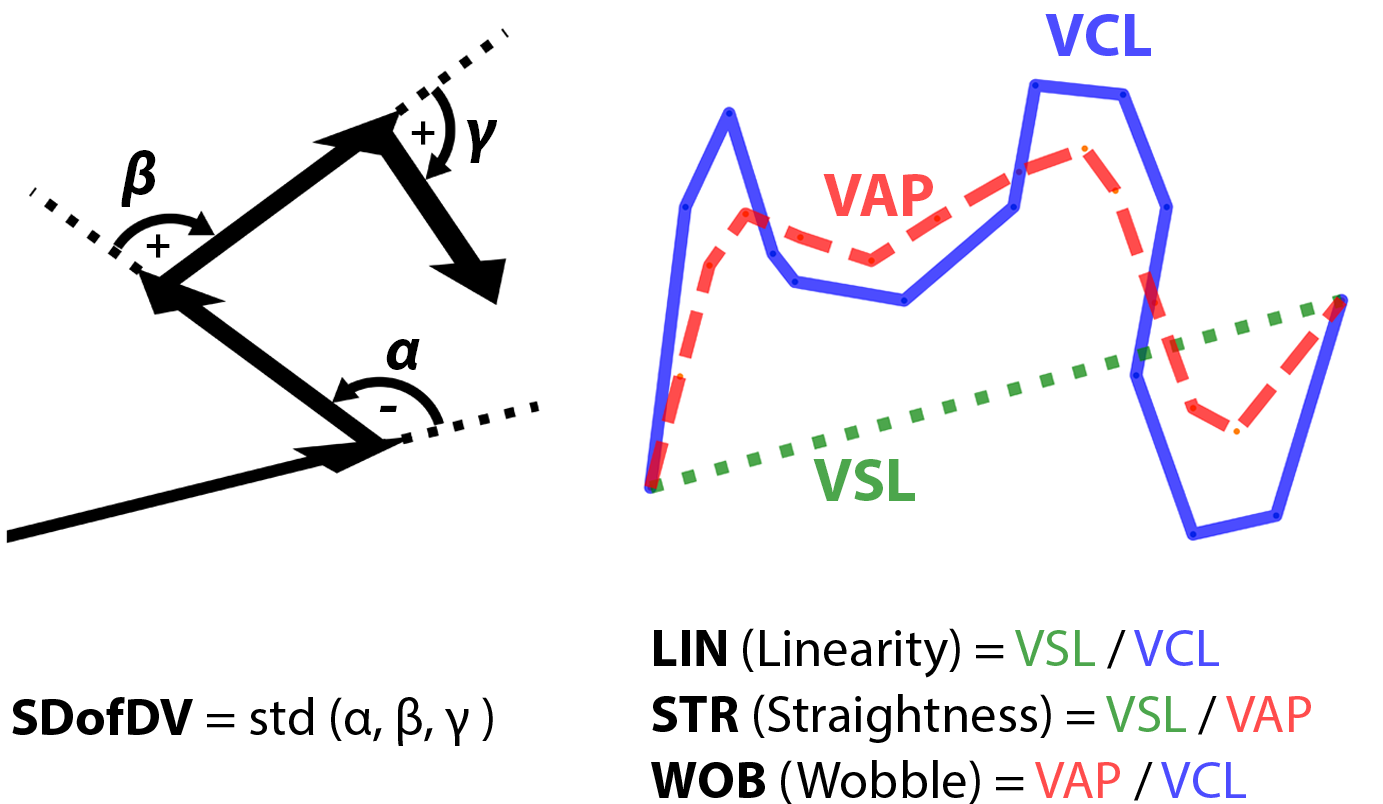
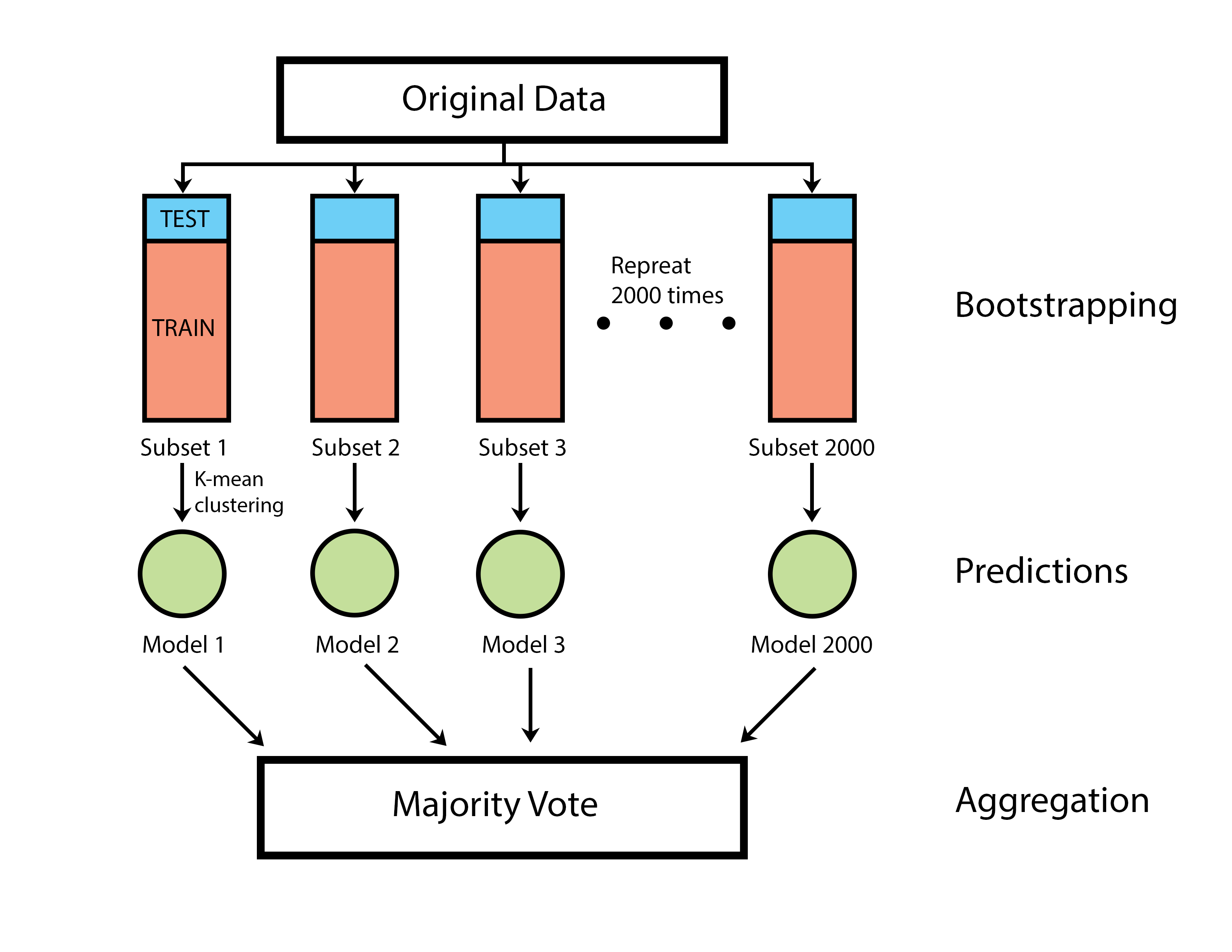
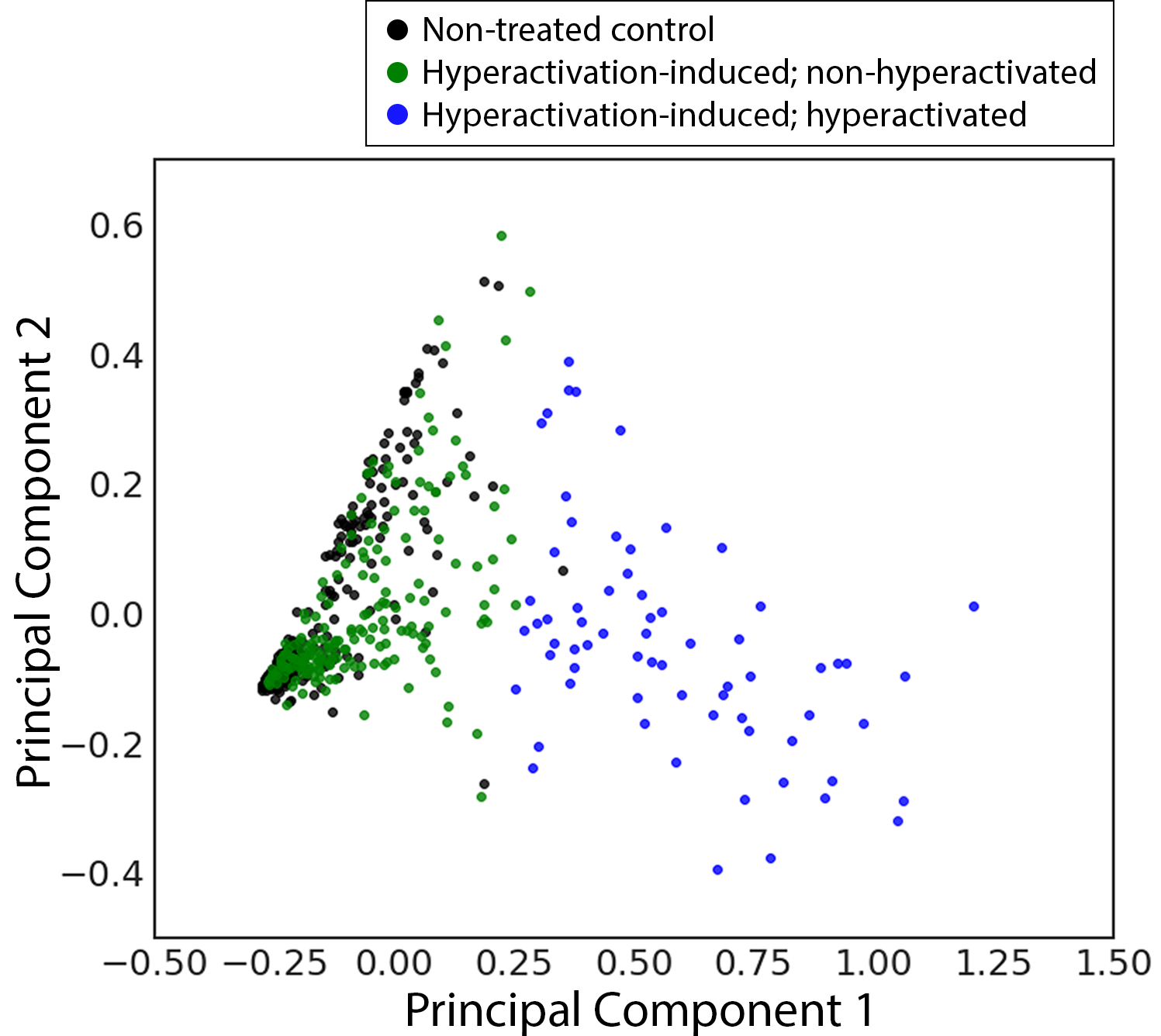
<-* Classification result shows a boundary between two classes
The unsupervised classification model divided trajectories in the hyperactivation-induced group into two classes. The green dots showed on the 2D PCA panel highly overlapped with the black dots. These spermatozoa did not undergo hyperactivation and their dynamics are similar to the non-treated control. In addition, the hyperactivation rate (Number of blue dots / Number of hyperactivation-induced spermatozoa) of the hyperactivation-induced group matches previously reported literature.
Before applying this model to in vivo trajectories, the model has to be validated by multiple viscoelastic environments which cover the range of viscosity of the fallopian tube in mouse. The model showed the consistency of performance over a wide range of viscosity. Therefore, it is ready to use to identify the hyperactivation state of in vivo spermatozoa.
* The consistency of performance in multiple viscosities ---->
Mediums were added methylcellulose at concentrations of 0%, 0.2%, 0.4%, and 0.6% (weight/volume), which represent viscosities of 1.0, 3.5, 10.5, and 29.4 (mPa•s), respectively. The kinematic viscosity of the medium was measured by a glass capillary. The hyperactivation rates of the hyperactivation-induced group with multiple viscosities were in the range of 19.5% and 23.7%, which were matched with the expected rates according to the previous literature.
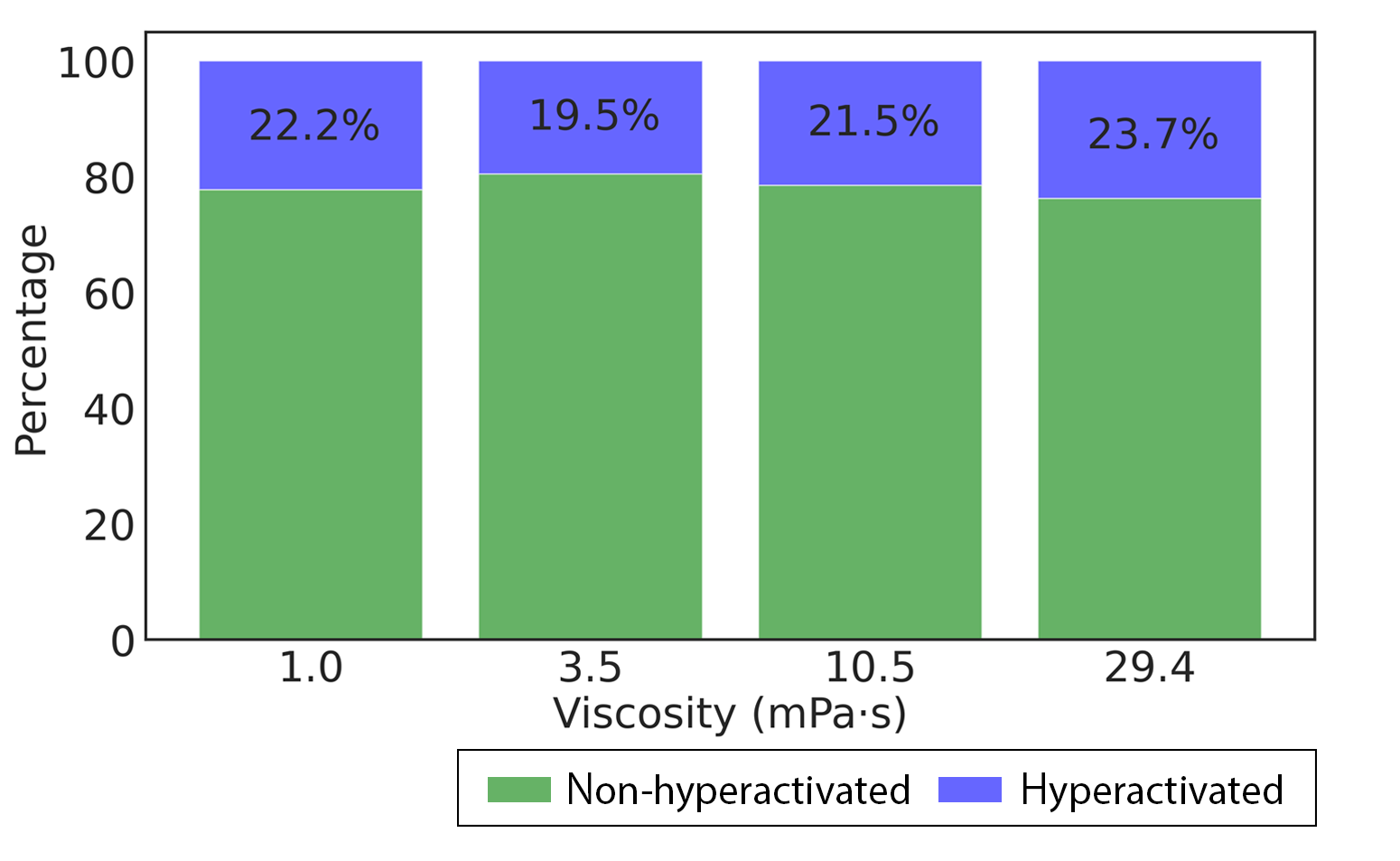
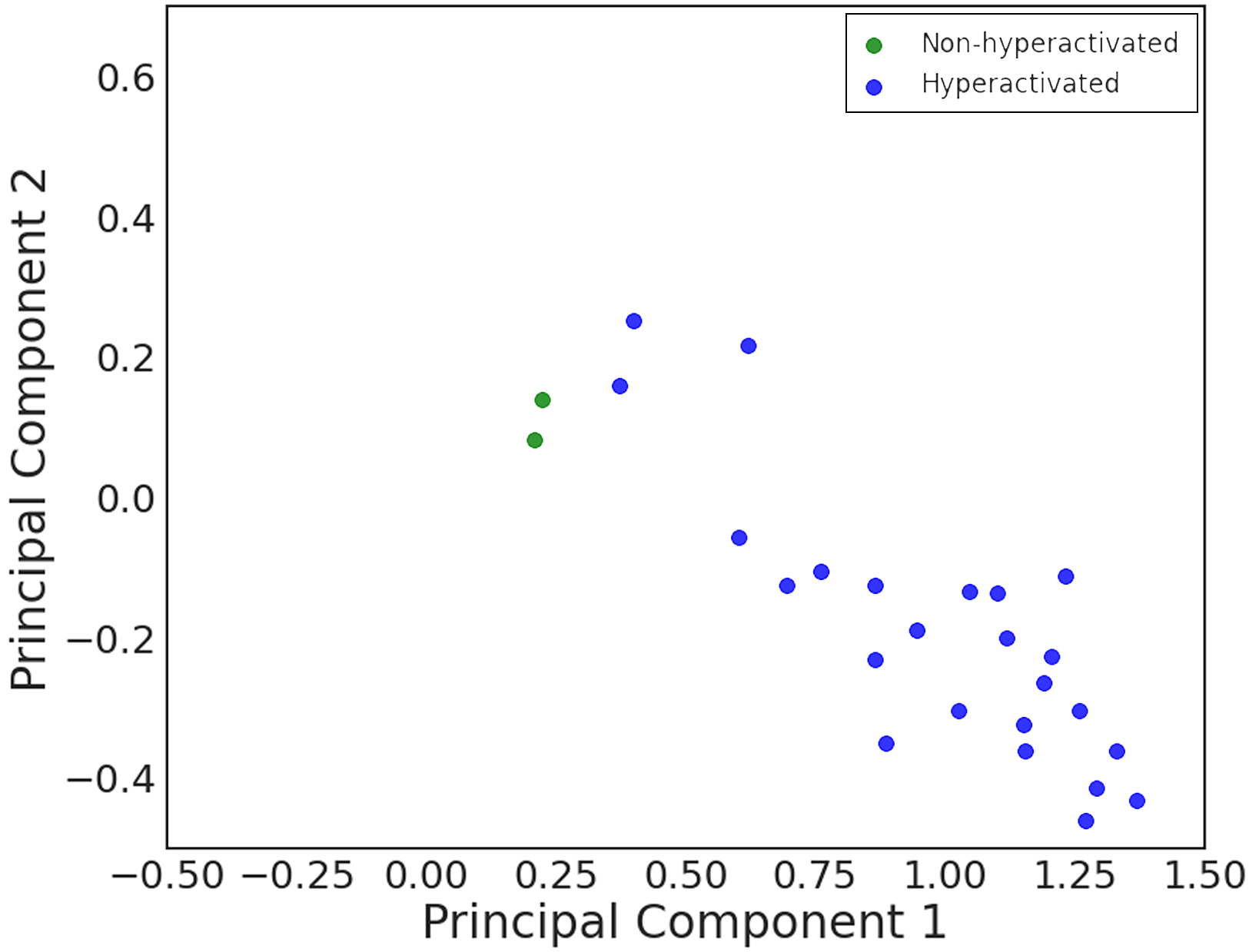
<----* In vivo trajectories analysis
in vivo trajectories were acquired by optical coherence tomography at the middle segment of the fallopian tube in the nearly natural state. The middle segment of the fallopian tube, the ampulla, was considered the site where fertilization occurs. Therefore, previous reports suggested that spermatozoa in this position are hyperactivated spermatozoa. Utilizing our unique imaging technology, we successfully acquired the movement of spermatozoa in the fallopian tube and evaluated them with the established classification model. Our data stated that 92% of spermatozoa in this area were identified as hyperactivated. The remaining non-hyperactivated spermatozoa were located close to the boundary at the 2D PCA panel.
Conclusion:
This project brings out an innovative quantitative approach to identify the spermatozoa hyperactivation state in vivo. The classification model showed consistent performance over a wide range of viscosity. Investigating the dynamics of the hyperactivated spermatozoa in traditional molecular methods is extremely difficult and time-consuming. Our study provides a unique perspective to investigate the dynamics of spermatozoa in vivo and has the potential to apply to clinical therapy and future research.